How Thinking in LAYERS
Avoids the Cluster Trap
Thinking in LAYERSÂ uses supply and demand within a system of trade area layers to model consumer buying behaviour across multiple trade areas. It doesn't double-count consumer spending, and therefore, metrics can be measured and compared across vastly different trade areas. It means no more over-competitive retail clusters. It finds the opportunities hidden by current location methods and ensures none are missed across Canada. This leads to optimized retail networks with higher revenue and lower costs.
1. Trade Area Model
Building a Digital Twin retail analytics model effectively means being able to explain the market: explain consumer shopping choice (demand side) inside the profitability of the current retail landscape (supply side). It why it's driven by a mountain of supply and demand data, and the product of a decade of research and development.
We call it Thinking in LAYERS - its how science deconstructs and then solves the toughest problems on the planet and its how we solved the real-life complexity of consumer shopping behaviour. Based on a decade of research and development, it's derived from Reilly's Law of Retail Gravitation - simply that consumers will travel longer distrances to larger retail centres that offer a greater selection of goods and services. This means that the complexity of consumer shopping choice can be deconstructed into unique trade area types. Thinking in Layers estimates seven unique overlapping trade area layer types (Figure 1). Each trade area type from the largest "Super Regional" to the smallest "Small Neighbourhood" shopping centres are uniquely defined and categorized.
These trade area layers effectively simplify the complexity of consumer shopping behaviour by deconstructing each type of shopping trip as a unique trade area type. Consumers choose the retail offerings in a trade area type based on the trade-off between convenience and the size of the retail offering. The larger the retail offering, the larger the trade area type and the more consumers are willing to be inconvenienced and vice versa. The choice to shop a particular kind of shopping centre represents a travel pattern that defines a unique trade area type that can be intelligently measured and accurately predicted.
Multiple choices create multiple trade area layers. The list of consumer choices effectively builds a natural shopping path for each consumer household as they purchase goods and services from different trade area types. Layers that roll up, so that convenience stores with more locations, for example, have small local trade areas and jewelry with less locations have large regional trade areas. Its the brilliance of Thinking in Layers, realistic trade areas for all retail store categories inside one system of trade area layers...for every household and retailer in Canada.
Figures 2 illustrates how trade area types are used to build a natural retail shopping path for households. The brown trade area is classified as a Small Community trade area called Yonge Eglinton Centre. Note how the region in the middle of the trade area is overlapped by higher-order trade areas, including a Large Community (green), Regional (blue), and Super Regional (black) trade areas. Households in this region will naturally migrate from Yonge Eglinton Centre to the SmartCentres Leaside trade area (green line) if the goods and services are not conveniently available, and then to the Manulife Centre (blue line), and then to the shopping district of Lawrence Allen and Yorkdale Shopping Centre, respectively. Shopping districts are a common occurrence in many larger shopping centres, where there is a mall (Yorkdale) and a power centre (Lawrence Allen) that make up the trade area.
Figure 1: 7 Shopping Centre Layers
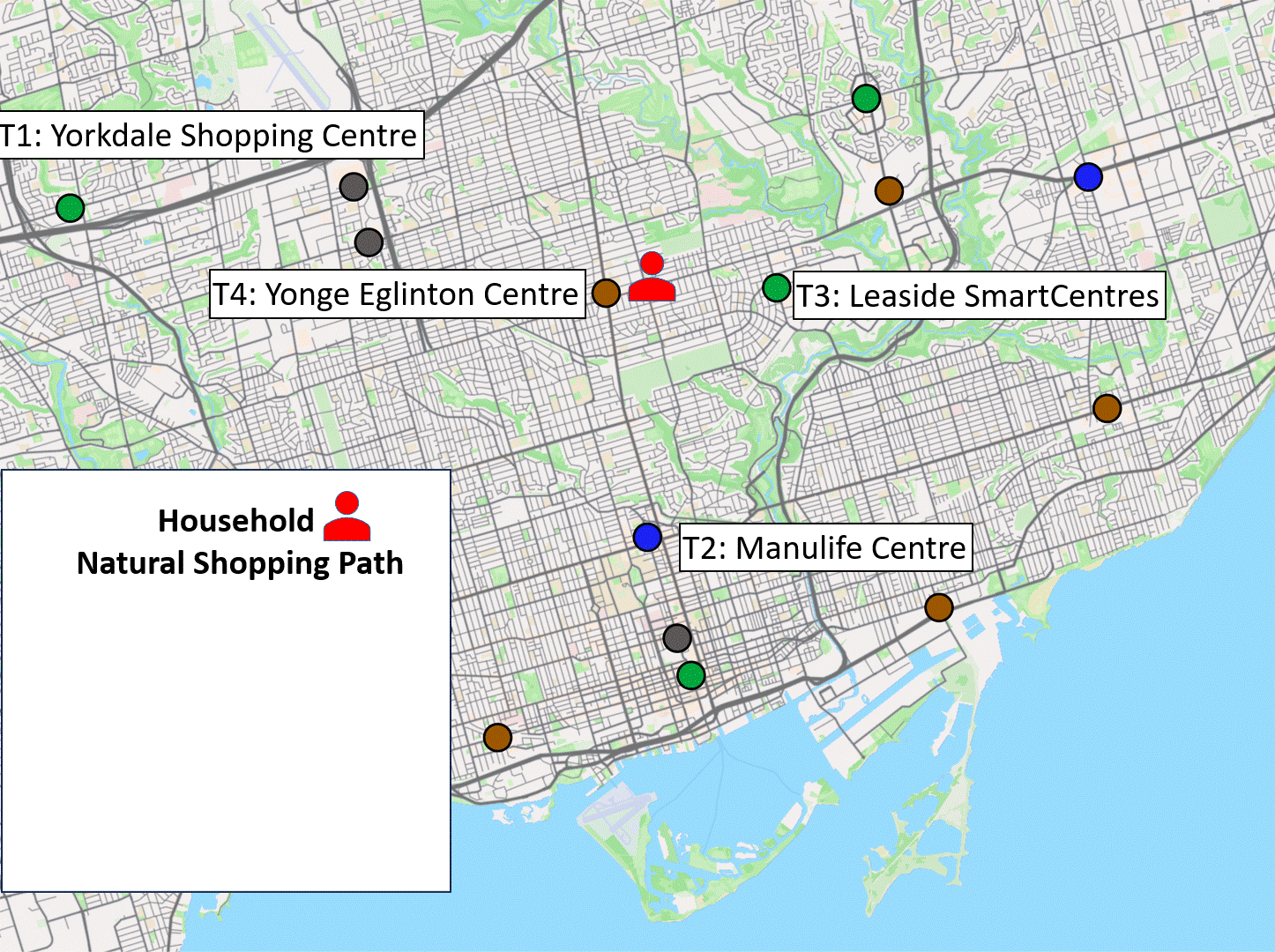
Figure 2: 7 Natural Shopping Paths
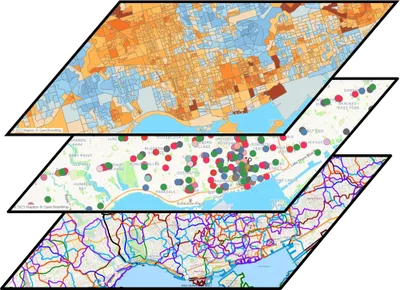
2. Supply and Demand Data
The framework of 7 layers provides the context to measure both supply and demand data for each individual trade area. Without both supply and demand data, you can't accurately measure the opportunity and, therefore, can't avoid the retail cluster trap. Figure 2 illustrates the demand, supply and trade area layers for the Toronto region.
Demand: We model our own consumer spend, wealth and demographic data for Canada. We specialize in modelling data to ensure its accuracy and completeness, drawing on decades of economics, statistical and mathematical model-building experience. We license our data to clients independently of our custom services and solutions. Please see our data products for more details.
Supply: We start Google Maps, the most complete business listing source in the world. We then clean, enhance and augment it to include the size of the business in some cases. This is critical to the evaluation of competition and the overall accuracy of Thinking in Layers. Competitor data is then grouped into about 300 retail categories. We license our data to clients independently of our location intelligence solutions. Please see our data products for more detail.
3. Model Calibration
Calibration is what ultimately gives Thinking in LAYERS its accuracy...and therefore its power. Calibration is possible only when the entire Canadian market is modeled so potential leakages and surpluses outside each household's natural shopping path are eliminated. It's the fundamental problem with conventional methods that treat trade areas in isolation from each other.
The calibration process effectively simulates the consumer's choice to shop at each potential shopping centre in their natural shopping path based on the value proposition of retail competitors. For example, an individual consumer makes the decision on where to purchase clothing based on the size and strength of clothing retailers in their natural shopping path of clothing retailers.
Advanced equilibrium modeling techniques are then used to estimate consumers' share of wallet, and therefore consumer spend in each of their potential shopping destinations based on the relative strength of each retail offering. This effectively reconciles retailers (supply) with consumer spend (demand) while ensuring that consumer spend is never double counted. This produces more accurate estimates of consumer spend and therefore more accurate estimates of the real retail opportunity. It's a new level of accuracy in location intelligence that conventional methods simply can't match!
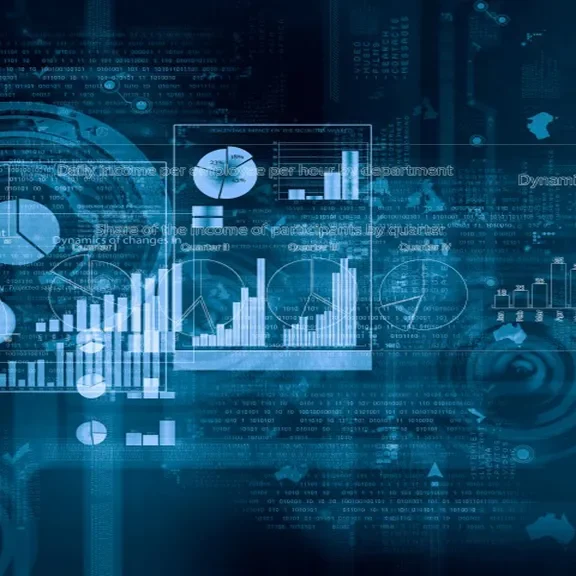
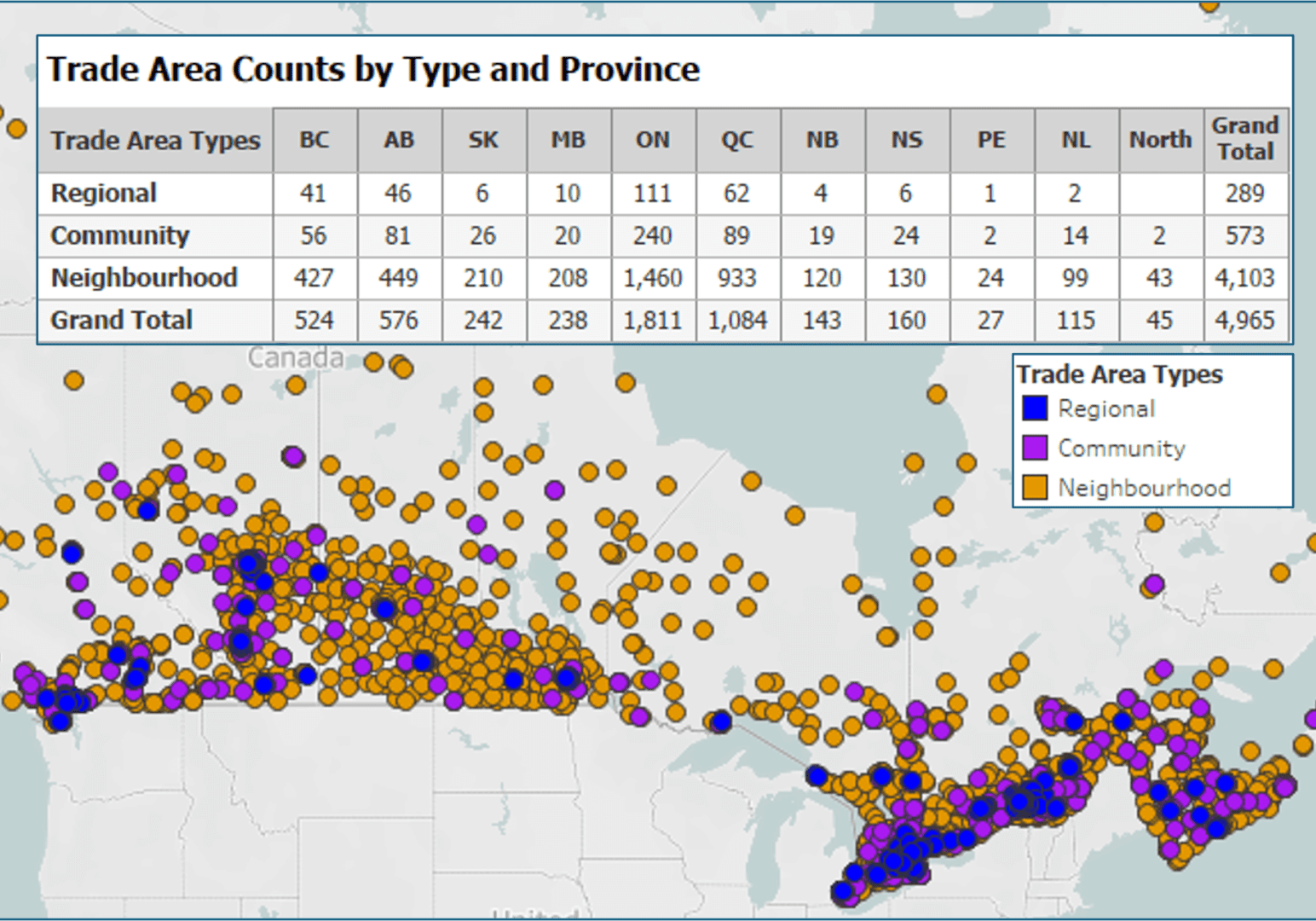
4. Location Analytics
Thinking in LAYERSÂ creates the foundation for true location optimization because it means we can now accurately compare retail opportunities for site across vastly different markets, regardless of the size or complexity of the market. It also goes beyond traditional retail gap analysis with its unique ability to score opportunities across retail store categories. Discover why certain retail business types have the best opportunity for success in a specific location, while others don't!
- Trade area type - categorizes all retail stores in Canada into 7 unique types of shopping centres: Super Regional, Regional, Large Community, Small Community, Large Neighbourhood, Medium Neighbourhood and Small Neighbourhood.
- Competitive analysis (supply side) - compares and scores the strength of the combined competitors in a trade area across almost 5,000 trade areas in Canada.
- Migration of consumers in/out of a trade area - quantifies the net number of consumers expected to migrate into and out of the trade area.
- Consumer spend analysis (demand side) - compares and scores the size of consumer spend after the migration of consumers into and out of the trade area.
- Retail opportunity analysis - combines the competitive and consumer spend analysis to estimate, score and rank the performance and opportunity across almost 5,000 trade areas in Canada and over almost 200 retail store type categories. Its how we guarantee to find the best opportunities, while ensuring none are missed...and without stumbling into over-competitive retail cluster traps.
5. Data Enrichment
A system of realistic trade areas also provides higher accuracy and context for additional data and analysis. These are factors that influence the process, but often target only a portion of the market, where should be incorporated at the end of the process. This ensures that micro factors don't overtake the macro supply and demand picture. We offer the following data:
- Demographic profile - a full set of demographic variables including population, population projections, income, income projections, family, households, dwellings, mobility, visible minority, employment, labour force, occupation, education, study field, commuting, religion, mother tongue, home language, immigration, and ethnic origin. Go to data dictionary
- Consumer spend - 300 variables including food, shelter, household operations, household furnishings & equipment, clothing, transportation, health care, personal care, recreation, home entertainment, education, smoking, alcohol, cannabis and more. Go to data dictionary
- Wealth metrics - over 50 household assets, debt and networth variables. Go to data dictionary
- Brand metrics and analysis - Measuring and tracking the health and performance of a brand is vital to understanding potential risks and opportunities for site specific sales growth. For more information
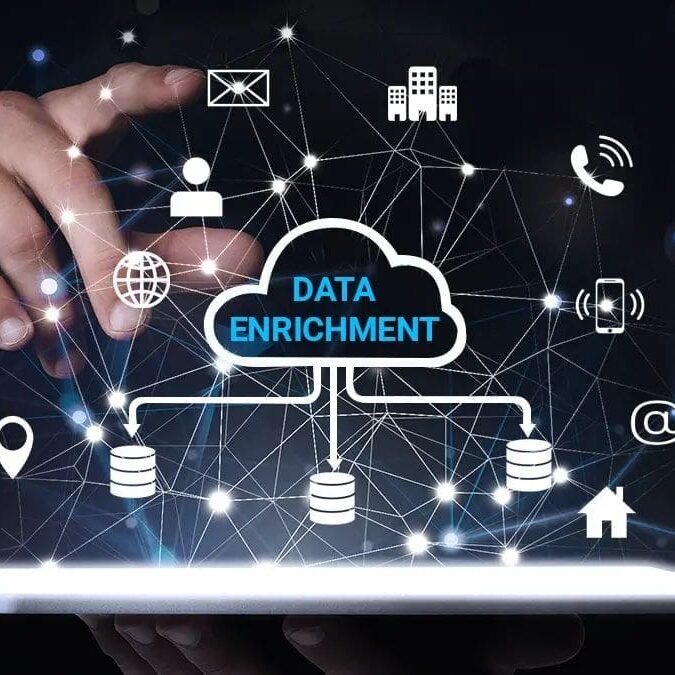